An account on AIES-2022
What happens if you put philosophers and computer scientists in the same room for two and a half days?
This is not the beginning of a lame joke but the premise of the Artificial Intelligence, Ethics, and Society conference.
The recent leap in the development of AI and its tenacious conquest of more and more industries brought AI Ethics into the forefront of interest for many AI specialists and philosophers. Traditionally, these two communities didn’t communicate with each other very often, but as AI keeps interweaving with more and more aspects of our daily lives, questions of our values, societal biases and incentives called for an urgency for bringing ethical debates closer to program specifications.
This year, I had the chance to attend AIES, which turned out to be a special island where these two communities met up to discuss their latest thoughts on the risks of AI and its current and potential future consequences.
I’ll give a short overview of the conference themes and will share some statistics on the university / company and country representations at the conference, pursuing the question:
Are we biased while talking bias?
Spoiler alert: probably (see last section for the details)
I will give my high level account of the conference, which probably won’t be comprehensive. I aim to give a short update on where the community stands and hope to motivate the reader to browse the proceedings. See a word cloud of the accepted paper titles and abstracts below, as a teaser.
Four main themes were discussed during the presentations and poster sessions:
- AI Auditing / Regulation
- Alignment and Decision making
- Explainability
- Social bias in AI systems
Let’s get into the details after a photo of the conference venue, the beautiful Keble College in Oxford.
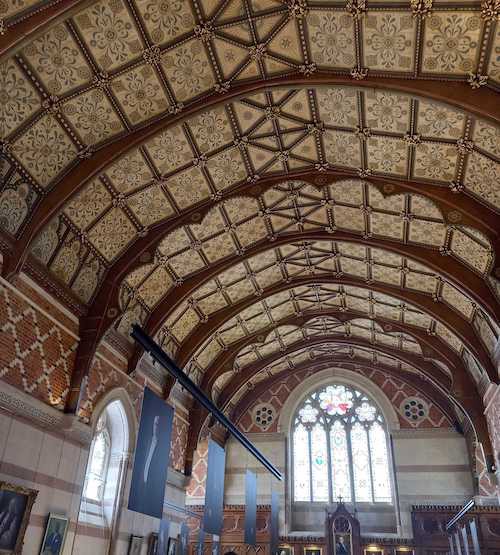
Auditing / Regulation
Legislation of AI is slowly developing in several countries, however, many AI practitioners, sociologists, philosophers and activists agree that it’s way too slow compared to the pace of AI development and industry application. Furthermore, the industry is already preparing for the upcoming legislations. Therefore, auditing AI companies and algorithms has become a central topic in AI Ethics.
Some talks touched on fairness alignment with regulation in Europe and in the US. Others analysed the stakeholders (AI experts, non-expert users etc.) of AI systems.
Deborah Raji, in her opening keynote talk, introduced taxonomies of audits and their pros and cons.
Initially, audits were sorted into three categories, first, second and third party audits (see table below).

Raji suggested to move on to a binary categorisation, based on the level of access to information and the level of independence. In this framework we talk about:
- Internal audits with direct access to algorithms and data but lack of independence from the organisation being audited.
- External audits which are performed by independent organisations but only have indirect access to algorithm outputs and data.
External audit
The role of external audit can be summarised in the following activities:
- They benchmark algorithms and data for bias
- They have access to the target audience and incentive to take them into account
- They can induce public pressure
- They often face hostile corporate reaction (Facebook goes after auditors, some companies introduce paywalls). But their actions have also managed to inspire them to improve their models! E.g., After the gender shades project several companies improved their algorithms.
Several useful audit tools from Raji’s talk included:
- Audit Accountability Tooling: e.g., Incident Reporting Systems: Artificial Intelligence Incident Database (AID)
- Audit Evaluation Tooling: e.g., Model cards, Data sheets etc.
The full list can be found here.
Internal audit
Internal auditing has the great potential due to the direct access to data and algorithms. However, several problems arise regarding market incentives, which prevent companies to do the audits or to publish the results (e.g., HireVue hired ORCAA for auditing but they restricted the information flow and the publication of results).
Some talks involved suggestions on policies to incentivise internal auditing, such as penalty default. Others analysed AI startup internal auditing attitudes [Bessen et al., Winecoff et al.]. Auditing frameworks have also been introduced to aid internal audits once there is an incentive to do so [Cachel et al., Raji et al.].
While being critical, Raji et al. writes:
Ultimately, internal audits complement external accountability, generating artefacts or transparent information that third parties can use for
external auditing, or even end-user communication. [Raji et al.]
What’s next
Raji’s suggestion for the next steps are:
- Further audit design
- Legal & institutional design
- Collective action: creating standards, communication
- Open Source Audit Tooling
Alignment and Decision making
Several philosophy and engineering talks were related to aligning human values with AI technology and its (future) consequences.
As I understand it, aligning human values and AI systems faces two immense challenges:
- Even though humans design AI algorithm objectives, model results can easily produce unwanted side-effects that we cannot control for. Such as data and model bias (see following section)
- People often do not agree on values and it has always been a challenge to formulate them.
Disclaimer: While I have a lay person’s interest in philosophy, my background is more in Computer Science, therefore my account of this area of the conference will definitely be less accurate than the others. I’d be more than happy to receive corrections and amendments in the comments or in private message.
Some topics that were addressed included:
- How social media affects self-concepts, including the observation that people have a desire for more transparency of these platforms.
- AI advisors (e.g., recommendations) cannot follow people’s moral changes. While predictive information changes human decision making.
- Ronald Arkin’s invited talk introduced studying robotic deception as a tool to understand human thinking.
- More talks touched on the long term AI benefits (strengthening communication, scientific discoveries) and risks (warfare, unaligned AI, exacerbating inequality)[Clarke et al., Bucknall et al.].
Karen Levy’s invited talk explored the state of US truck drivers, one of the major groups which can be affected by AI, i.e, self-driving cars. It turns out that high tech surveillance is a more immediate threat to them than self-driving trucks taking their jobs.
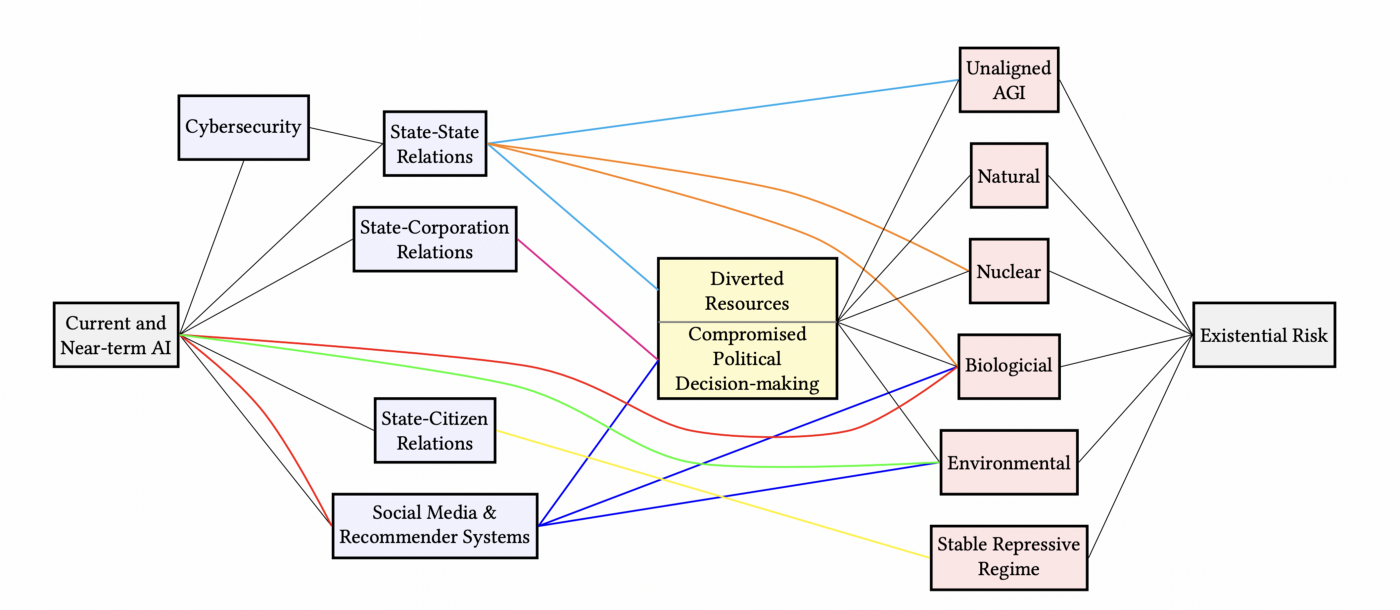
Shannon Vallor in her invited talk embedded our conversations in the framework of treating AI as a mirror of us and our society. She inspired us to try to avoid losing ourselves in it, and instead, use it as an opportunity to recognise our apparent faults and make amends.
Explainability
Recent, widely used AI models’ decision making process is almost impossible to directly interpret for a human, which implies huge risks. This problem is addressed by the Explainable AI community.
Explainability came up in the context of human — AI collaboration and decision making, and bias in Explainable AI design. Some showed that leakage of prior bias in AI explanations can lead to confirmation bias during decision making.
Suggestions for improving decision making included counterfactual explanations [Dai et al., Sate] and dialogue-based explanation with reasoning.
One context where the need for explainable AI is most tangible is social media platforms where a lack of transparency has been shown. Explainability can also be a tool for bias detection.
Bias / Fairness
AI systems both reflect and can amplify our collective biases coming from their training data. It has been pointed out that besides having biased data, AI models can also amplify bias by how they handle data distributions. Consequently, many conference talks focused on social bias in data and in the models, predominantly regarding race and gender.
Model bias
It has been shown that biases equating American identity with being White are learned by language and-image AI, and propagate to downstream applications.
Gender bias in word embeddings brings social bias reflected in data in the spotlight.
Dataset bias
Some papers measured the role of dataset features and annotations in terms of bias.
Failed attempts for debiasing
One of the strongest conclusions of the conference was that naively removing simple gender and race attributes does not debias algorithms. This has been supported by several corresponding studies:
Removing gender and race from recruitment systems doesn’t help with mitigating bias. Attempts to “strip” gender and race from AI systems often misunderstand what gender and race are, casting them as isolatable attributes rather than broader systems. Furthermore, the attempted outsourcing of “diversity work” to AI-powered hiring tools may unintentionally entrench cultures of inequality and discrimination by failing to address the systemic problems within organisations.
Various other papers came to similar conclusions. For example, models can identify patient self-reported race from clinical notes even when the notes are stripped of explicit indicators of race. Others presented that merely removing demographic features from ad recommendation algorithm inputs can fail to prevent biased outputs; and ignoring the protected attribute alone does not fix biased financial models.
One of the few works by authors with industry associations came from LinkedIn on recommendation system biases and feedback loop effects. They found that although seemingly fair in aggregate, common exposure and utility parity interventions fail to mitigate amplification of biases in the long term.
Proposed solutions
Some solution proposals include contrastive counterfactual fairness, discovering complex forms of bias through other seemingly unrelated attributes using evolutionary algorithms and two-distribution hypothesis test.
Open questions
The AI bias literature mostly focuses on race and gender, however, there is a considerable room for improvement on the questions we ask.
Firstly, I have barely seen work which deals with non-binary genders, which may be due to the fact that it is easier to find strong signals for binary categories.
Secondly, my impression is that the discourse of race and social bias in general is very US centric. I haven’t seen much work on ethnic groups more prevalent in other parts of the world (e.g., roma people in Eastern Europe). There is also very little effort in discovering features (with some exceptions) instead of starting from our prior assumptions. This, in my opinion, has the risk of trying to force the global discourse on social groups and their problems into the one narrow narrative.

Bias in talking bias?
I though it would be interesting to see what parts of the world were represented at this conference, where so many people are involved in fighting our biases. The talks and the conversations sparked from them were immensely enlightening and enjoyable. My intention with the statistics below is to put these conversations into perspective, in order to make us further brainstorm on how to improve; a piece of constructive criticism.
Most authors came from academia. Out of the 125 talks, 6 were on papers by authors with company associations. Since AI became a part of our everyday lives mostly through industry applications, I would be happy to see more of them joining the discussion on AI Ethics. As a result, I was glad to listen to the small minority of authors who were associated with the following companies: IBM, LinkedIn, Upturn, SCHUFA, Microsoft and Arthur AI.
In order to investigate locations, I visualised the distribution of organisations represented at conference talks, and their country of origin. Note, that I used the country of the universities / companies, not the country of origin of the authors themselves, as I did not have data for that.
The figure below shows that there was a substantial dominance of the US and the UK in the discussion. The rest of the organisations mostly came from Western Europe. Other continents (except for Japan, Singapore and India were not represented.)
There is an even more striking US dominance in the “general” lightning talks sections — excluding student talks (see below).
The student talks were more “European”, however, Eastern Europe (with the exception of Serbia) and the rest of the world (except for Singapore) weren’t represented.
Four invited speakers came from US universities (Berkeley, Georgia Tech, Brown, Cornell), one from Edinburgh, UK.
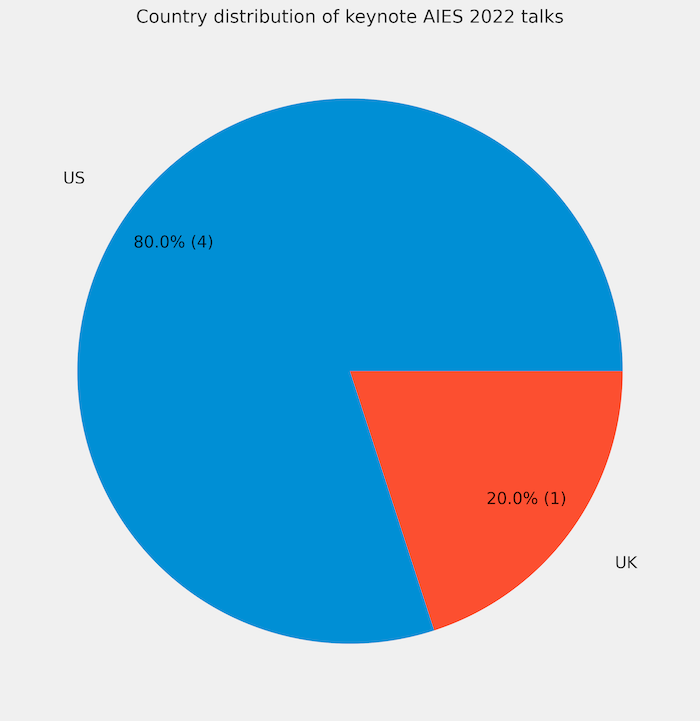
Finally, I visualised the representation of countries on maps (all talks, regular + invited talks and student talks respectively).
Method, data and code
The data and code for generating these figures are all available on Github. Please let me know if you find any mistake! Feel free to contribute to the repo if you feel like it.
GitHub - anitavero/aies2022: Statistics of the AIES 2022 conference.
You can't perform that action at this time. You signed in with another tab or window. You signed out in another tab or…
github.com
Dataset
I manually created tables of all lightning talks and the countries of the organisations. The statistics above include all authors’ affiliations, not just the one who presented the talk.
Conclusion
I personally found AIES-2022 very enlightening and a lot of fun.
Many important topics have been raised, discussed and several supporting statistics made the findings stronger.
I will definitely read more on the various proposals and suggestions that were mentioned during the talks, in order to make my own work in AI more conscious.
With the above statistics, I was trying to shed light on other meta biases we may have in this community, which is very much involved in ethics and bias. Although, I did not have data for that, despite the imbalance in organisation locations, my impression is that the background of the authors / speakers was more diverse. The dominance of the US and Western Europe in research and tech is not a new finding. I think it is somewhat mitigated by people coming from different parts of the world to do research at these places. Nevertheless, the environment we are in affects us, thus taking into account these biases might be useful.
The conversations at this conference touched upon very important topics, and I hope to see it continuing in the future!