The policy landscape
In some quarters, it has become an accepted fact that content moderation on social media platforms is politically biased, and laws have been drafted and passed in opposition to this. In the US, State laws in Texas and Florida (both likely to be reviewed by the Supreme Court next year) have included “must-carry” provisions banning moderation based on viewpoint discrimination and for political and media organizations respectively; in the UK, the proposed Online Safety Bill includes special protections against moderation actions for news journalism posts.
Why have these concerns become so prevalent? A 2018 study examined the varying “folk theories” that users arrive at when they do not understand why action was taken against their post or account, and these often come down to assumptions of bias. Accordingly, there has been movement towards better explanation of content moderation decisions, including in the Santa Clara principles. The EU’s newly-operative Digital Services Act will require that moderation notifications sent to impacted users explain the decisions—and that they disclose any use of automated moderation.
The increasing use of automated moderation may reassure users that the actions taken on a platform are less prone to human bias—regardless of the realities of limited personal discretion in commercial human content moderation and the problems of AI bias. While keyword filtering has been in use for many years and and hash-matching is core to fighting the spread of child sexual abuse imagery, developments in natural language processing and machine learning have enabled new levels of sophistication and breadth in automated content moderation. Both large platforms and third-party providers (including Unitary AI) are constantly improving the capabilities of these systems.
There has been substantial research into how people perceive the decisions made by algorithms in different contexts, such as hiring processes and the legal system, but in the last year and a half, several studies have been published that specifically investigated how users perceive automated decision making in content moderation. Three studies are summarized below, focussing on their results relevant to the practical questions facing platforms about what to tell their users about their use of AI in content moderation decisions.
The studies
Study 1: Christina A. Pan et al., “Comparing the Perceived Legitimacy of Content Moderation Processes: Contractors, Algorithms, Expert Panels, and Digital Juries” (April 2022)
Goals
This study sought to establish how users perceive different forms of content moderation decision-making (including automated), primarily drawing criteria from prior research into how citizens view the legitimacy of courts: trustworthiness, fairness, and jurisdictional validity.
Method
100 US Facebook users, recruited via Amazon Mechanical Turk, were presented with “decisions” on 4 of 9 real Facebook posts that were potentially violating in 3 different policy categories, together with a moderation source: contractors, algorithms, expert panels or user juries. They rated the decisions according to factors indicating legitimacy as well as whether they agreed with the outcome or endorsed the continued use of the source, and also gave some explanation. They were then asked directly about their views of the 4 moderation sources.
Results
Algorithmic moderation was perceived as the most impartial source, but was seen as more legitimate with human oversight. Algorithmic moderation was about equivalent to contractor moderation overall—more impartial, but less trustworthy. Those who did rate the algorithm highly tended to make the legitimacy conditional, e.g. on the ability to appeal the decision to a human. (The expert panel came out as most legitimate, with its expertise being the determining factor, as opposed to its independence.) Perhaps unsurprisingly, the most determinative factor in assessing legitimacy was whether the subject agreed with the decision! One notable user preference identified by the researchers was for multiple opinions to inform moderation decision making.
Study 2: Maria D. Molina & S. Shyam Sundar, “When AI moderates online content: effects of human collaboration and interactive transparency on user trust” (May 2022)
Goals
Molina and Sundar explored how people think about AI decision making: (1) do they use a “positive machine heuristic” assuming objectivity and consistency or a negative one where computers are seen as unable to understand human emotion and complexity, and (2) are people concerned about a lack of human agency or involvement when algorithms make decisions alone. The study probed how these perspectives impacted understanding of AI content moderation, how transparency can help, and how all of these factors impact trust in the system.
Method
676 US-based participant, recruited via Amazon Mechanical Turk, were shown one of 4 posts that possibly violated hate speech or suicidal ideation policies, along with the policies themselves. The possible conditions were: source (AI / human / both), classification (flagged / not flagged), and transparency (none / list of violation-indicative keywords shown / list shown with opportunity to suggest additional keywords). They then were surveyed on their trust in the system, their perceived degree of understanding of it, and agreement with the outcome. Their perspectives on how they think about AI systems were also measured.
![AI source of classification and flagged classification decision [left]. Human source of classification and not flagged classification decision [right]. The condition where AI and human provide a decision together had both visual avatars together.](https://cdn.prod.website-files.com/675823350267b290d7ca1c88/675823350267b290d7ca1f21_ZP-_R7Wzfw5TX5q4AqzsF0rpkdJa2LgTnr_FgcsPd53JtaPx2HP3RRSX51iVamSjnQ1Kan_1y-auveddrZ4O8Tg44gowA0ls3_wy07Tmo2rfTTWrcwiKZq9qW97Dff8-QeLJW4aj68rpnheUIfU_WC8.png)
Source: Maria D. Molina & S. Shyam Sundar.
Results
Users who invoked the positive machine heuristic had greater trust in AI moderation, and the converse was true for the negative heuristic. Ideas about human agency appeared to have little impact, but where the users themselves were able to participate by giving feedback on the keyword lists, or when AI and humans made the moderation decision, they trusted the system more. Transparency was correlated with more trust and perceived understanding. On the whole, human moderators were not trusted any more than AI moderation.
Study 3: Marie Ozanne et al., “Shall AI moderators be made visible? Perception of accountability and trust in moderation systems on social media platforms” (July 2022)
Goals
Aware of the potential costs of transparency in moderation, this study assessed what benefits may also accrue in disclosing when algorithms are responsible for decisions. The investigators selected metrics across three aspects of content moderation (decision, process, and system) to provide contextual data for the key measures of trust and accountability. Focussing on the policy category of harassment, they also examined how more or less ambiguity as to if a violation occurred impacted user perceptions.
Method
This project made use of a simulated social media platform including other dummy users. 397 US-based participants, recruited via Amazon Mechanical Turk, set up accounts, created posts, and logged in at least twice a day over two days. They encountered one feed item each day with a moderated comment, a link to the harassment policy, and a question as to whether they agreed with the moderation decision. Each post was described as being flagged by an automated system, other users (as might occur on Reddit, Discord or a Facebook group), or no identified source. After the two days, a questionnaire assessed their perceptions of the moderation decisions (agreement / trust / perceived fairness), process (understanding), and system (perceived objectivity and accountability, i.e. acting in users’ best interests).
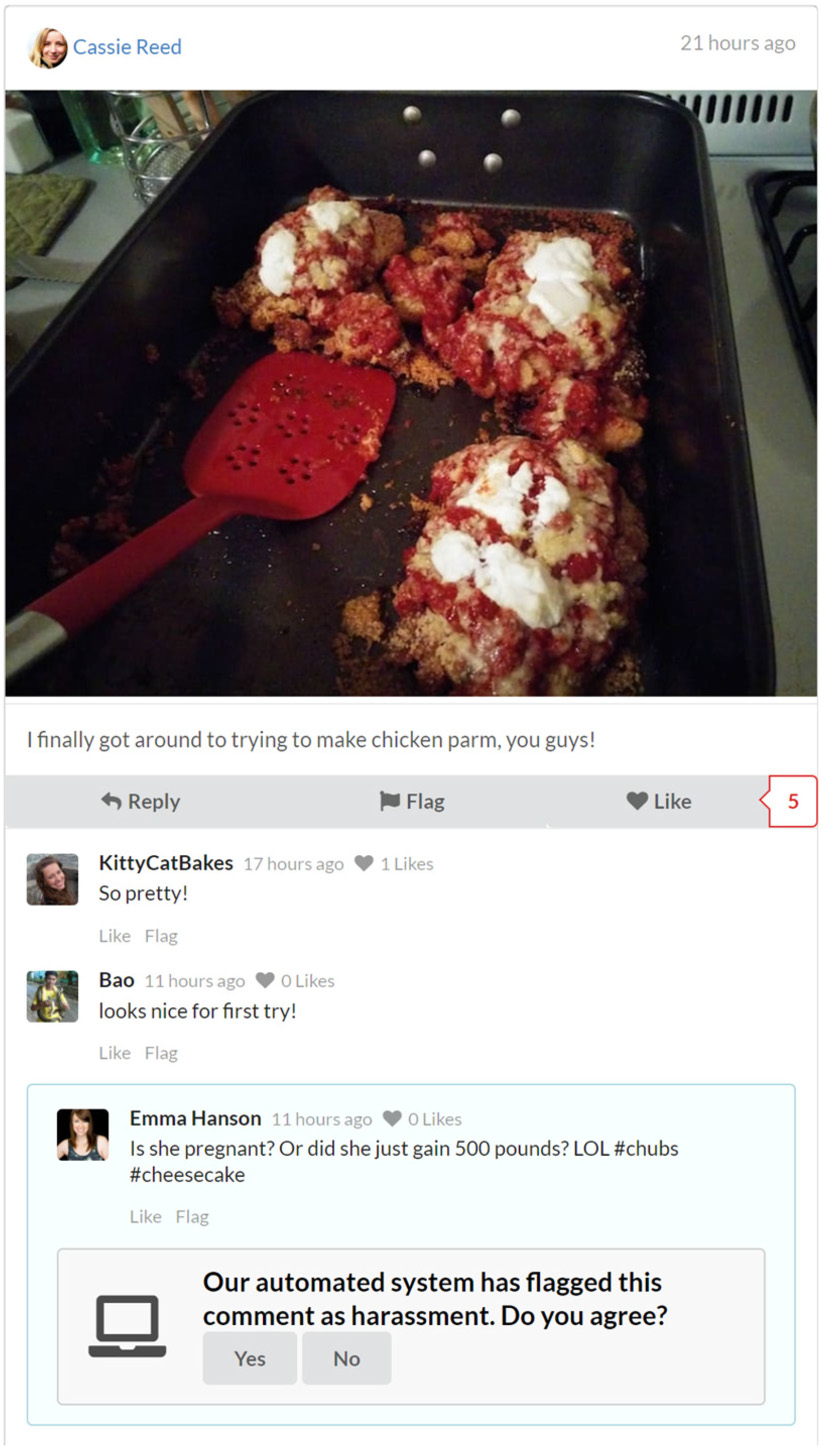
Results
The AI moderator was trusted less than other users, but on a par with an unidentified moderation source. Perceptions of fairness were similar across all three sources, as were levels of agreement with moderation decisions and perceived understanding of how moderation works. Perhaps the most interesting finding was that the automated moderator was perceived as less accountable only when the content was ambiguously violative. The authors surmise: “people may be willing to yield to machine judgments in clear-cut and unequivocal cases, but any kind of contextual ambiguity resurfaces underlying questions about the AI moderation system because of a possibility of errors and questions about whose interests and values it reflects.”
Takeaways
Two caveats on applying the findings of these studies: First, all of these studies analysed the perspective of bystanders to the content moderation process. Though this may be of direct use to those working in public policy or high-level operational decisions, for those designing feedback for individual users, it does not take into account emotional reactions to being subject to a moderation action oneself (Pan et al.’s finding that agreement with the decision outcome being the most important factor in determining user impressions is instructive here!). Second, they each compared AI moderation to other options, which differed from study to study. The real—or imagined—alternatives on any individual platform may play a role in user perceptions of automated moderation.
Regardless of the findings of these studies, it is a best practice to give users insight into how moderation works on a platform for legal, principled, and pragmatic reasons. However, it is also worthwhile to consider how to explain the workings of the system, including the use of AI, in order to cultivate trust and goodwill. Takeaways from the studies that can inform how to frame content moderation decisions that use AI include:
- A significant proportion of users are willing to trust automated moderation under certain conditions (such human oversight / escalation). (Pan et al.)
- Users place trust in expertise and multiple opinions (Pan et al.), which both play a role in the training of classifiers.
- People have both positive and negative preconceptions about automated systems (Molina & Sundar) and it may be possible to draw attention to the positive traits.
- Transparency into the details of the classifier’s operation can result in greater trust. (Molina & Sundar)
- Users may be more willing to see AI moderation decisions in a positive light for some kinds of content, such as clear-cut policy violations. (Ozanne et al.)
Expanding on this last point, Ozanne et al. chose harassment as the policy category for the study because it tends to require more context, and therefore it may seem less likely to lend itself to effective AI moderation. Taking this together with insights from Molina & Sundar, we might consider what aspects of computer abilities could be emphasized for actions on different content types, e.g. objectivity for misinformation and consistency for hate speech. Additionally, if user responses are based in peoples’ preconceptions about automated decision making, there may be significant differences across geographic or other user segments, and as trends develop (such as the bad press that other social media algorithms have received), each with their own risks and opportunities for sympathetic explanations of AI moderation.
None of these researchers were able to make use of the scale and continuity of live platforms. However, their work can inform starting points for iterative experimentation that can provide stronger and more fine-grained evidence for what kinds of explanations result in different perceptions of moderation systems, as well as future on-platform behaviors. This can be one more building block in the development of satisfied user bases, efficient content moderation systems, and safer platforms.
If you are interested in learning more about how users perceive content policies, read our interview with former TikTok Policy Manager, Marika Tedroff.